Coming soon from
Easy expense management and reporting to save you time, money, and sanity
Toggl Work, a smart expense management and reporting tool, is coming soon to a browser tab near you.
Get notified when we launch:
Reasons to get excited about Toggl Work:
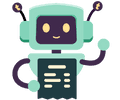
AI-pumped efficiency
Bid adieu to manual data entry. With Toggl Work, you’ll enjoy accurate and fast receipt processing — even with low-quality or handwritten uploads. And by fast, we mean processing and auto-filling receipt information in under 7 seconds.
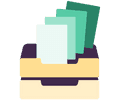
Any expense, handled
From contractor invoices and travel expenses to subscriptions and benefits — Toggl Work will help you efficiently manage any expense. But not just manage — automatically categorize and file under correct cost centre policies to keep it clean and efficient.
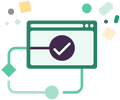
Intelligent automations
Avoid inconsistent expense reporting, late submissions or non-compliant expenses with a host of intelligent automations. Set budget rules for expense categories and cost centres, automate approvals and set up time-sensitive reminders.
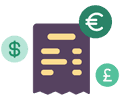
Real-time transparency
Avoid currency confusion with real-time conversion support for over 170 currencies. And enjoy real-time translations to English or other selected languages.
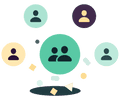
Simple tracking and reporting
Keep stakeholders in the loop and your expense budget on track with presentable dashboards and configurable expense management processes.
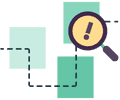
Detailed expense audit trails
Record all expense transactions happening in your organization — from expense creation to reimbursement — to stay compliant, transparent and audit-ready.
Seamless integrations with:
+ MORE COMING SOON!