The Toggl Blog
Learn how to increase project profitability and grow your business with our guides and tips.
Latest posts…
Who is Toggl anyway?
Toggl is a set of productivity tools built with stress management in mind. We’ve been around the block for more than 10 years and we’re serious about remote working and better work management.
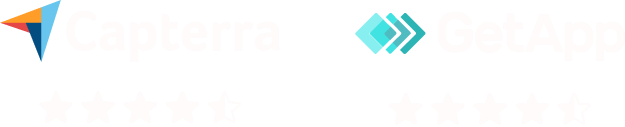
Project Management…
can get really tricky. And we’ve got some tricks!
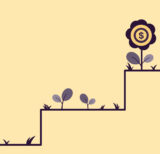
Project budget management is the process of planning, allocating,…
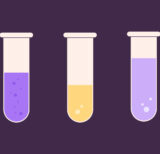
Achieve optimal resource utilization. Boost project profits and team…
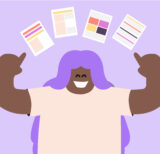
Find out how to create a successful marketing plan,…
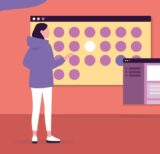
Learn about project management basics, methodologies, roles, responsibilities &…
Business Growth
We love growth and money too, let’s talk about it!
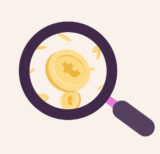
Are you tired of constantly struggling to increase your…
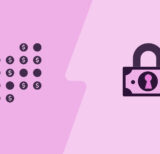
Time and materials vs. fixed fee – which type…
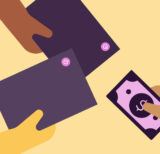
Chocolate or vanilla? Red pill or blue pill? Flat…
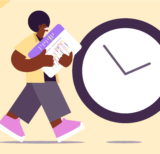
Timesheets have long been touted as the go-to method…
Talent Acquisition…
is no easy feat. But we’ve got ideas for you!
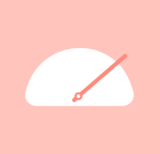
Hiring is tough, and with the rise of remote…
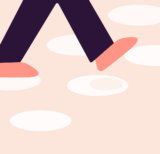
71% of companies lack a formal employee offboarding process,…
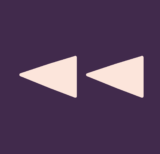
Why wait for candidates to come to you when…
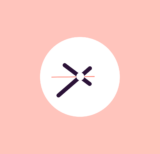
Henry Ford is world-famous for his innovative methods, his…